Aman Khawaja discusses how AI is revolutionising the structure of employment, considering short- and long-term effects and how regulation and policy could be used to mitigate challenges and maximise opportunities.
In this essay, I will examine how AI is reshaping employment structures, and potential short and long-term shifts in the labour market. I shall explore AI’s benefits and challenges, delving into its multifaceted relationship with the labour market, and ultimately conclude that its overall effect is unknown but predicted to be broadly neutral. Lastly, I shall propose regulatory and policy measures aimed at mitigating challenges and maximising opportunities from AI.
How is AI revolutionising the structure of employment?
To begin, I shall explore the literature surrounding the benefits that AI could have on the labour market. AI can create new job opportunities, particularly high-skilled jobs, and complement workers to increase productivity (Noy and Zhang, 2023; Choudhury et al., 2020). AI can automate repetitive tasks, allowing workers to prioritise complex tasks (Zarifhonarvar, 2023). Prescott (2023) stated that by 2035 AI could boost UK labour market productivity by 25%. In 2022, Acemoglu et al. conducted a study about AI’s impact on labour markets. Using firm-level data on US online vacancies from 2010 to 2018, they found rapid growth in AI-related vacancies. However, the impact of AI-labour substitution on employment is currently too small to investigate (Ibid). Moreover, the literature supports the coexistence of worker and workplace AI (Jaiswal et al., 2021; Wilson and Daugherty, 2019). Li et al. (2019) contend that businesses should incorporate new technologies which support, not replace, workers to boost productivity and profit, evidencing firms’ commitment to protecting their workforce. I shall now use the UK as an example economy that could greatly benefit from AI adoption. Currently, the UK has a tight labour market, with unemployment rates at 4.2% nearing a record low and economic inactivity rates rising to 20.8% (ONS, 2023). The Government’s 2023 Spring Budget announced multiple employment incentives, evidencing the political priority devoted to boosting labour productivity. Therefore, the government working alongside businesses to adopt AI will reap numerous advantages.
On the other hand, introducing AI into business operations can alter the composition of the labour market by differentiating workers by abilities (Lebovitz et al., 2022; Allen and Choudhury, 2022; Acemoglu et al., 2022). The rise of AI exacerbates inequalities as technology usually tends to skilled workers; this is skill-biased technological change (SBTC). New technologies, e.g. robotics and automation, often require a higher level of skill to operate and maintain. Consequently, there is increased demand for high-skilled and higher-educated workers, with negligible effect on unskilled workers, generating job polarisation (Dell and Marlar, 2020; Acemoglu, 1998). Subsequently, there is potential for existing earning differentials to widen more, exacerbating income inequality (PwC, 2021). Figure 1 depicts how individuals lacking qualifications will likely experience net job losses, and the opposite for highly educated people:
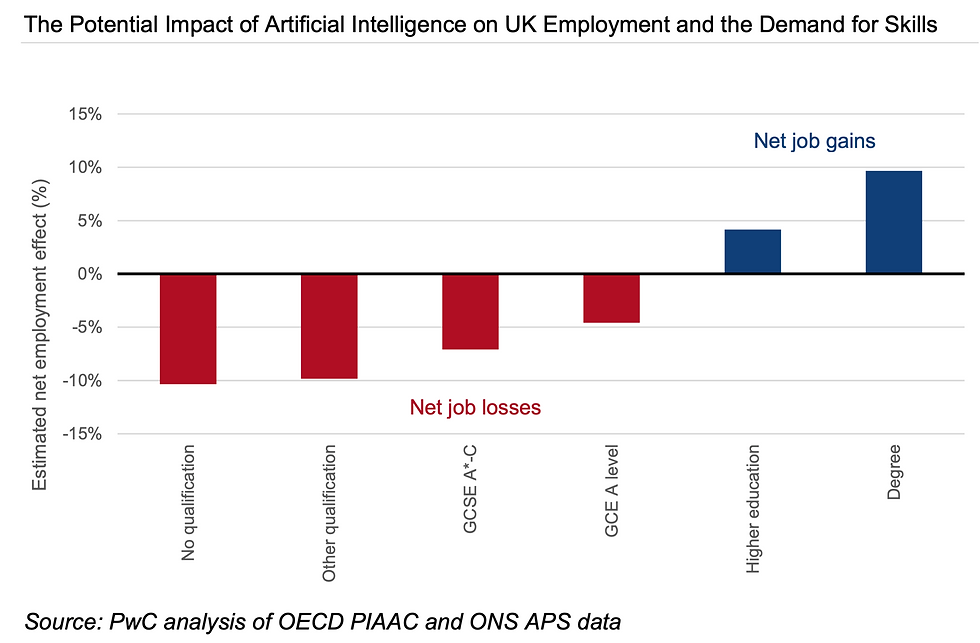
Figure 1: PwC (2021)
There is evidence of SBTC being common among the US, the UK and other advanced economies since the 1980s (Berman et al., 2018). Therefore, the literature surrounding the impact of AI on the labour market is complex and different workers face different consequences.
Potential short-term labour market shifts
In the short-term, AI will likely substitute labour-intensive tasks with automation. This can result in job losses and earnings decreased (Frontier Economics, 2018). Bughin et al. (2018) undertook a study and discovered that approximately 50% of all work activities could be automated with current technology. Moreover, PwC (2021) estimates that 7% of UK jobs could be displaced over the next 5 years, equivalent to 2.2 million jobs. There will be a potential temporary misalignment between the skills employers desire for adapting technologies, and those which employees can offer. This may reduce demand for labour and give rise to unemployment, although the labour market will likely stabilise as people develop new skills. As depicted in Figure 2, there is an inelastic labour supply - assuming labour supply remains constant in the short-run due to the long training and education time required for reskilling workers - reduced labour demand, and wage reduction:
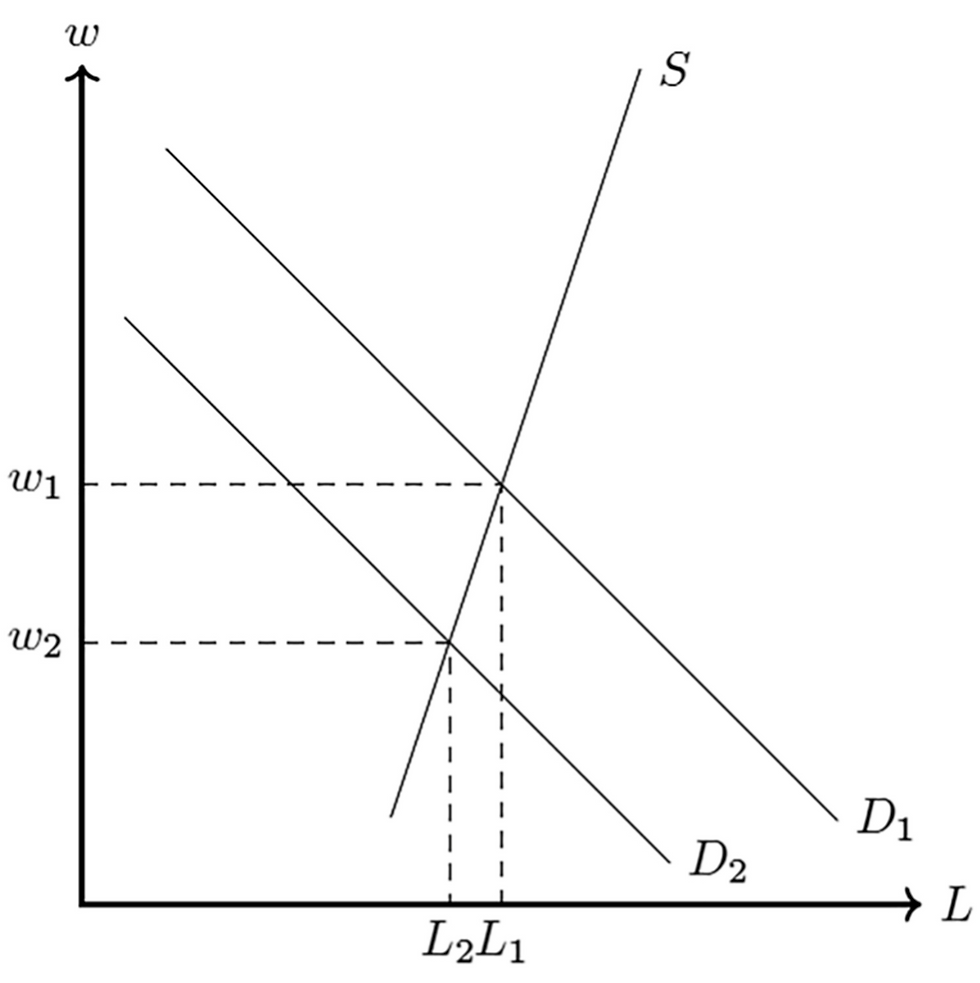
Figure 2
However, this is partially offset by the productivity effect. Caselli and Manning (2017) and Acemoglu and Restrepo (2018) propose models of an economy where final goods are produced using labour and capital inputs, and new technologies (AI) allow multiple labour tasks to be automated, demonstrating how AI substitutes and augments labour. Subsequently, goods and services, produced by automation, either increase in quality or are cheaper. Hence, consumers demand more products, so demand for labour increases. But, in the short-term, the substitution outweighs the productivity effect (Ibid). Therefore, the dynamics of short-term labour market are nuanced as they depend on the intricate balance between the substitution effect, productivity effect and creation of new jobs.
I shall now use ChatGPT to examine and exemplify the short-term effects of AI on employment. ChatGPT is a LLM, containing text prediction and generation as some of its features. Hui et al. (2023) adopted a difference-in-differences research design and data from Upwork, to investigate how the introduction of generative AI affected freelancers in writing-related jobs. They concluded that freelancers experienced a 2% decrease in the number of monthly jobs and a significant 5.2% deduction in monthly earnings. This is displayed in Figure 3, demonstrating the effect of text-based generative AI on freelancers’ employment:
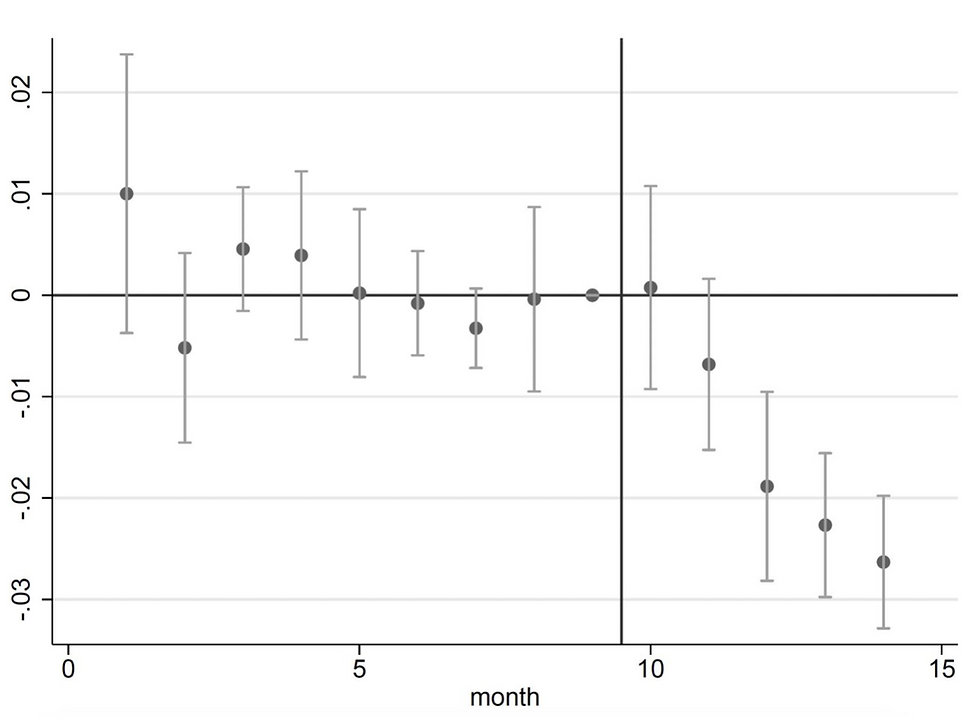
Figure 3
These results align with previous studies undertaken by Eloundou et al. (2023) and anecdotal evidence. Thus, the ChatGPT example accentuates the real-world consequences of AI on short-term labour market dynamics by evidencing the substantial adverse effects on employment.
Potential long-term labour market shifts
In the long-term, the productivity effect will likely strengthen and fully offset the substitution effect: the previous short-term reduction in demand for labour (Frontier Economics, 2018). This is due to the capital accumulation effect. Unlike the short run, capital stock is not fixed and more machines can be produced, which is necessary as automation boosts demand for capital (e.g. robots). Consequently, the costs of capital and production drop, hence the productivity effect becomes stronger (Ibid). Therefore, in the long-term, AI makes up for initial job displacements by creating new jobs. However, job creation by AI is complex as it differs significantly by industry. This is due to differences in the estimated growth of demand for different goods and services, and the different extent of labour-augmenting AI across industries (PwC, 2021). Figure 4 graphically represents the estimated net employment effects of AI on a variety of UK industries over the next 20 years:
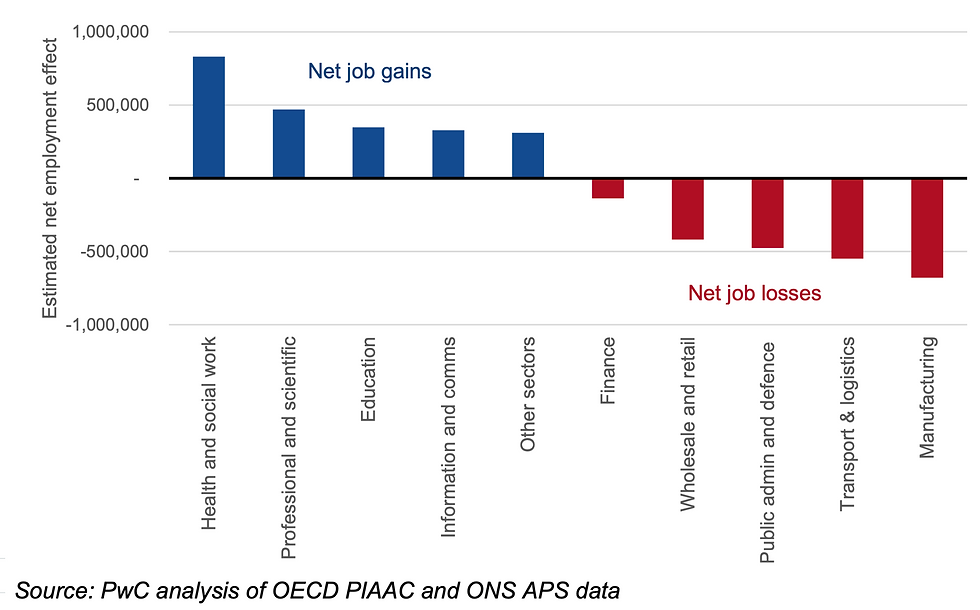
Figure 4: PwC (2021)
The health and social work sector has the largest estimated net job gains from AI. Due to the UK’s ageing population and health being classified as a ‘superior good’, as income levels rise, demand for health services will boost massively. AI can streamline tasks and increase productivity in the health industry, for example, automating the analysis of medical images for diagnoses. But, compared to sectors like manufacturing, AI is likely to be more successful by complementing and augmenting workers within health services, rather than substituting them. Overall, the long-term net effect of AI on employment is nuanced and currently unclear, but the most plausible assumption is a neutral effect.
Potential regulation and policy responses
With the development and innovation of AI, policymakers face a serious challenge as they must foster progress and maximise opportunities, whilst simultaneously mitigating against adverse impacts that could arise for workers and consumers. Thus, I shall propose a range of potential policy responses to consider.
Firstly, education is pivotal in driving AI adoption and combatting inequality. Policymakers could consider teaching key concepts around technology, such as AI and potential ethical issues, providing students with fundamental knowledge to become digital citizens (Crafts et al., 2018). As students pursue higher education, there should be specialised topics about new technologies and AI within STEM subjects to increase numbers of AI specialists.
Secondly, policymakers can employ policies to support low-income workers and individuals that may have suffered from a job displacement or loss due to AI. These could include: universal basic income programs, tax reforms, and redistributive mechanisms that transfer wealth generated by AI capital to individuals whose jobs were affected by AI (Bughin et al., 2018).
Lastly, policymakers must ensure that benefits of AI implementation are distributed throughout society and not concentrated amongst the wealthy or in one region. These initiatives could consist of: giving AI adoption advice to businesses of all sizes, promoting diversity and inclusion in AI development and investing in AI infrastructure in underserved communities (Crafts et al., 2018). Thus, these potential policy responses will allow policymakers to mitigate challenges while maximising opportunities from AI.
In conclusion, the impact of AI on the labour market is multifaceted; it possesses the challenges of job displacement in the short-term and exacerbating inequalities between workers with different education levels due to SBTC. However, there is the long-term potential for AI to create opportunities, boost productivity, and foster economic growth. Hence, nuanced policy responses are required to address the evolving landscape of AI, mitigating challenges, and maximising opportunities for a sustainable and inclusive future.
Essay by Aman Khawaja
Bibliography
Acemoglu, D. (1998). ‘Why Do New Technologies Complement Skills? Directed Technical Change and Wage Inequality’. Quarterly Journal of Economics, 113(4), pp. 1055 – 1089.
Acemoglu, D. and Restrepo, P. (2018). Artificial Intelligence, Automation and Work. SSRN Electronic Journal, pp. 197 – 236.
Acemoglu, D., Autor, D., Hazell, J., and Restrepo, P. (2022). ‘Artificial intelligence and jobs: Evidence from online vacancies’. Journal of Labor Economics, 40(S1), S293 – S340.
Allen, R. and Choudhury, P. (2021). ‘Algorithm-Augmented Work and Domain Experience: The Countervailing Forces of Ability and Aversion’. Organization Science, 33(1), pp. 149 – 169.
Berman, E., Bound, J. and Machin, S. (1998). ‘Implications of Skill-Biased Technological Change: International Evidence’. The Quarterly Journal of Economics, 113(4), pp. 1245 – 1279.
Bughin, J., Seong, J., Manyika, J., Chui, M. and Joshi, R. (2018). ‘NOTES FROM THE AI FRONTIER: MODELING THE IMPACT OF AI ON THE WORLD ECONOMY’. [online] McKinsey Global Institute. Available at: https://www.mckinsey.com/~/media/McKinsey/ Featured%20Insights/Artificial%20Intelligence/ Notes%20from%20the%20frontier%20Modeling%20the%20impact%20of%20AI%20on%20 the%20world%20economy/MGI-Notes-from-the-AI-frontier-Modeling-the-impact-of-AI-on[1]the-world-economy-September-2018.ashx. [Accessed 20 Jan. 2024].
Caselli, F. and Manning, A. (2017). ‘Robot Arithmetic: Can New Technology Harm All Workers or the Average Worker?’. [online] Centre for Economic Performance, London School of Economics. Available at: https://cep.lse.ac.uk/pubs/download/dp1497.pdf. [Accessed 24 Jan. 2024]
Choudhury, P., Starr, E. and Agarwal, R. (2020). ‘Machine learning and human capital complementarities: Experimental evidence on bias mitigation’. Strategic Management Journal, 41(8), pp. 1381 – 1411.
Crafts, N., Ghahramani, Z., McLean, A. and Wilson, A. (2018). ‘The impact of artificial intelligence on work’. [online] The British Academy. Available at: https:// www.thebritishacademy.ac.uk/documents/280/AI-and-work-evidence-synthesis.pdf. [Accessed 21 Jan. 2024].
Dell, K. and Marlar, G. (2020). ‘Assessing the Impact of New Technologies on the Labor Market: Key Constructs, Gaps, and Data Collection Strategies for the Bureau of Labor Statistics’. [online] U.S. Department of Labor Bureau of Labor Statistics. Available at: https://www.bls.gov/bls/congressional-reports/assessing-the-impact-of-new-technologies-on[1]the-labor-market.pdf. [Accessed 27 Jan. 2024].
Eloundou, T., Manning, S., Mishkin, P. and Rock, D. (2023). ‘GPTs are GPTs: An Early Look at the Labor Market Impact Potential of Large Language Models’. [online] arXiv:2303.10130 [cs, econ, q-fin]. Available at: https://arxiv.org/abs/2303.10130. [Accessed 23 Jan. 2024].
Frontier Economics. (2018). ‘THE IMPACT OF ARTIFICIAL INTELLIGENCE ON WORK: An evidence review prepared for the Royal Society and the British Academy’. Available at: https://royalsociety.org/-/media/policy/projects/ai-and-work/frontier-review-the[1]impact-of-AI-on-work.pdf. [Accessed 30 Jan. 2024].
Hui, X., Reshef, O. and Zhou, L. (2023). ‘Artificial intelligence and its short-term effects on employment’. [online] CEPR. Available at: https://cepr.org/voxeu/columns/artificial[1]intelligence-and-its-short-term-effects-employment#:~:text=Much. [Accessed 22 Jan. 2024].
Jaiswal, A., Arun, C. J. and Varma, A. (2021). ‘Rebooting employees: upskilling for artificial intelligence in multinational corporations’. The International Journal of Human Resource Management, 33(6), pp.1 – 30.
Lebovitz, S., Levina, N. and Lifshitz-Assaf, H. (2022). ‘Is AI Ground Truth Really True? The Dangers of Training and Evaluating AI Tools Based on Expertsʼ Know-What’. Management Information Systems Quarterly, 45(3), pp. 1501 – 1526.
Li, L., Li, G. and Chan, S. F. (2019). ‘Corporate responsibility for employees and service innovation performance in manufacturing transformation’. Career Development International, 24(6), pp. 580 – 595.
Noy, S. and Zhang, W. (2023). ‘Experimental Evidence on the Productivity Effects of Generative Artificial Intelligence’. [online] MIT Economics. Available at: https:// economics.mit.edu/sites/default/files/inline-files/Noy_Zhang_1.pdf. [Accessed 25 Jan. 2024].
ONS. (2023). ‘Unemployment - Office for National Statistics’. [online] www.ons.gov.uk. Available at: https://www.ons.gov.uk/employmentandlabourmarket/peoplenotinwork/ unemployment#:~:text=The%20alternative%20estimates%20for%20September. [Accessed 30 Jan. 2024].
Prescott, K. (2024). ‘The bots are coming, but will they take away our jobs?’ [online] www.thetimes.co.uk. Available at: https://www.thetimes.co.uk/article/the-bots-are-coming[1]but-will-they-take-away-our-jobs-bt23jlqzm. [Accessed 30 Jan. 2024].
PwC. (2021). ‘The Potential Impact of Artificial Intelligence on UK Employment and the Demand for Skills: A report by PwC for the Department for Business, Energy and Industrial Strategy’. [online] GOV.UK. Available at: https://assets.publishing.service.gov.uk/media/ 615d9a1ad3bf7f55fa92694a/impact-of-ai-on-jobs.pdf. [Accessed 29 Jan. 2024].
Wilson, H. J., Daugherty, P. R. (2019). ‘Creating the symbiotic AI workforce of the future’. MIT Sloan Management Review, 61(1), pp. 1 – 4.
Zarifhonarvar, A. (2023). ‘Economics of ChatGPT: A Labor Market View on the Occupational Impact of Artificial Intelligence’. SSRN Electronic Journal.
Comments